Best Practices for AI-Powered Scenario Planning
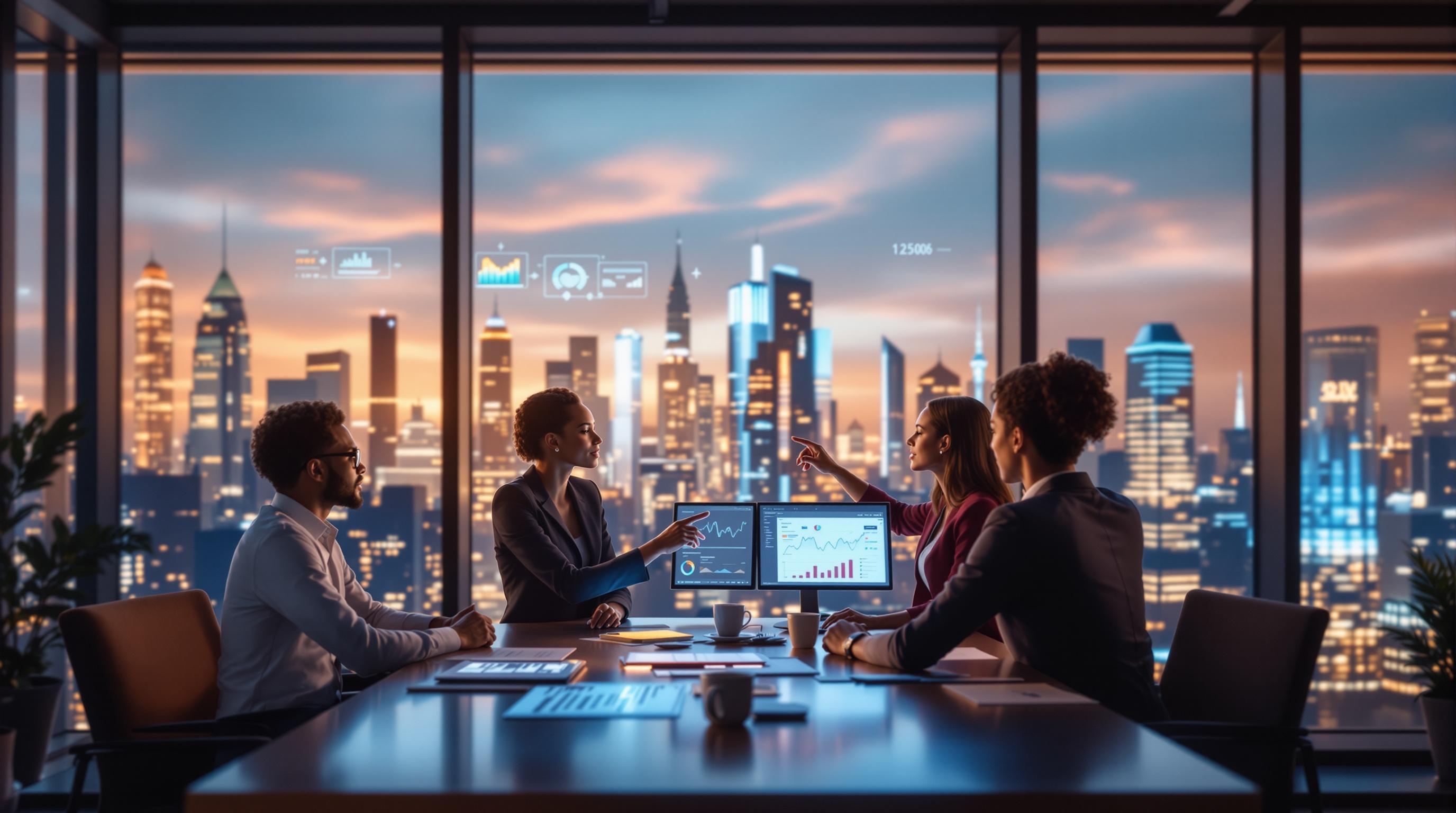
AI is transforming scenario planning, making it faster, more accurate, and better at predicting risks. By leveraging AI, businesses can analyze vast amounts of data, adjust forecasts in real time, and uncover trends that traditional methods miss. Here's what you need to know:
-
What is Scenario Planning?
It’s the process of preparing for different future outcomes by modeling various business and economic conditions. AI enhances this by analyzing more data and creating detailed, reliable forecasts. -
Key Benefits of AI in Scenario Planning:
- Detects hidden patterns in data
- Updates scenarios instantly with new information
- Processes thousands of variables simultaneously
-
Steps to Get Started:
- Gather high-quality historical data (financial, market, and operational metrics).
- Choose the right AI model (time series, regression, neural networks, etc.).
- Train and refine the model using structured processes.
- Build financial scenarios by focusing on critical drivers like customer metrics, costs, and market trends.
- Use AI insights for risk assessment and backup planning.
-
Practical Tips:
- Ensure data is complete, accurate, and updated regularly.
- Monitor model performance with metrics like MAPE and RMSE.
- Align AI-driven forecasts with your KPIs for better decision-making.
AI-powered scenario planning helps businesses adapt to market changes, spot risks early, and make smarter financial decisions. Start by preparing your data, selecting the right model, and integrating AI insights into your planning tools.
Data Preparation Steps
High-quality data is the foundation for effective AI scenario planning and reliable forecasts. Preparing this data is a key step before selecting and training AI models.
Historical Data You’ll Need
To create accurate AI-driven scenarios, you’ll need a solid collection of historical data across various business areas:
Financial Data
- Detailed revenue records
- Breakdown of fixed and variable costs
- Cash flow statements and balance sheets
- Profit and loss statements (at least 24 months of history)
Market Intelligence
- Industry growth rates and market share trends
- Competitor pricing and positioning
- Key economic indicators for your sector
- Customer acquisition costs and lifetime value metrics
Operational Metrics
- Production capacity and utilization rates
- Supply chain performance data
- Employee productivity statistics
- Patterns in resource allocation
Ensuring Data Quality
Maintaining high data quality is essential for reliable AI model performance. Here’s a breakdown of important quality criteria and how to meet them:
Quality Standard | What It Means | How to Achieve It |
---|---|---|
Completeness | All critical fields are filled with no missing values | Use automated validation tools |
Accuracy | Data reflects true business outcomes | Perform regular reconciliation |
Consistency | Uniform formatting across datasets | Implement standardized ETL processes |
Timeliness | Data is updated on a regular schedule | Set up automated pipelines |
Relevance | Data directly supports forecast goals | Conduct periodic audits |
To ensure these standards are met, automate as many validation processes as possible, assign clear ownership for datasets with documented procedures, and conduct monthly audits. Focus on identifying gaps in completeness, spotting errors, ensuring timely updates, and maintaining consistency.
Proper data preparation lays the groundwork for a successful AI model implementation.
AI Model Setup
Selecting AI Models
Choosing the right AI model for scenario planning starts with understanding your forecasting goals and the data you have available. Here’s a breakdown of common model types and their uses:
- Time Series Models: Best for short-term forecasts and identifying seasonal patterns. These models rely on consistent historical data to detect trends and cycles.
- Machine Learning Regression: Handles complex, non-linear relationships effectively, especially when diverse predictive features are available.
- Neural Networks: Suited for long-term analysis and recognizing intricate patterns, though they require a lot of data and processing power.
- Ensemble Methods: Combine outputs from multiple models to improve accuracy and assess risks more effectively.
When evaluating models, consider factors like the volume of your data, prediction timeframes, update frequency, computational resources, and how easily the model's results can be interpreted. Once you’ve chosen a model that fits your needs, focus on refining it through a structured training process.
Model Training Process
After selecting a model, optimizing its performance involves these key steps:
-
Initial Data Split
Separate your dataset into training, validation, and test subsets to ensure accurate evaluation. -
Feature Engineering
Develop indicators that reflect seasonality, growth trends, market dynamics, and risk factors. -
Model Validation
Use cross-validation, test under various economic scenarios, and compare results to a baseline model to reduce overfitting. -
Performance Monitoring
Track metrics like Mean Absolute Percentage Error (MAPE) and Root Mean Square Error (RMSE) for accuracy. Also, monitor bias and the reliability of prediction intervals. -
Continuous Improvement
Regularly retrain models with updated data, reassess feature importance, fine-tune hyperparameters, and document changes for transparency.
At Phoenix Strategy Group, we apply these detailed methods to help clients confidently navigate financial uncertainties through precise forecasting.
sbb-itb-e766981
Building Financial Scenarios
Key Financial Factors
Create AI-driven scenarios by zeroing in on critical financial drivers. Focus on areas like:
- Customer metrics: Customer acquisition costs (CAC), lifetime value (LTV), and conversion rates
- Cost structure: Fixed and variable costs, along with efficiency metrics
- Operational metrics: Cash conversion cycle, inventory turnover, and accounts receivable/payable
- Market dynamics: Interest rates, industry growth trends, and competitive factors
Tie these elements to financial outcomes for precise forecasting. Incorporate these drivers directly into your financial planning tools for better alignment and accuracy.
Financial Model Integration
Use reliable data and well-trained models to embed AI insights into your financial planning systems while maintaining data accuracy.
- Real-time Updates: Use APIs to sync AI models with planning tools for live data integration.
- KPI Alignment: Match AI-driven forecasts with your key performance indicators to improve decision-making.
Integration Level | Key Components | Benefits |
---|---|---|
Basic | Weekly KPIs, Revenue & Expense Tracking | Better visibility into performance |
Advanced | Forecasting & Budgeting, Cashflow Modeling | Enhanced decision-making support |
Enterprise | Integrated Financial Model, Data Engineering | Broader scenario analysis capabilities |
"As our fractional CFO, they accomplished more in six months than our last two full-time CFOs combined. If you're looking for unparalleled financial strategy and integration, hiring PSG is one of the best decisions you can make."
– David Darmstandler, Co-CEO, DataPath
Conduct weekly reviews of model outputs to refine assumptions and ensure scenarios remain actionable.
Using AI Insights
Risk Assessment
Turn AI-generated scenarios into practical strategies by focusing on measurable metrics that evaluate both likelihood and financial impact. Here are three key ways to assess the probability of scenarios:
- Confidence Scores: Keep an eye on AI model confidence levels, prioritizing scenarios with scores above 85%.
- Historical Validation: Compare predictions with past performance data to gauge accuracy.
- Variance Analysis: Measure differences between predicted and actual outcomes to refine forecasts.
To evaluate financial impacts effectively, use a structured framework based on time horizons:
Time Horizon | Key Risk Metrics | Response Triggers |
---|---|---|
Short-term (0-6 months) | Cash flow, Working capital | 15% deviation from baseline |
Medium-term (6-18 months) | Revenue growth, Margins | 20% shift in market conditions |
Long-term (18+ months) | Market share, Industry position | 25% change in growth trajectory |
This framework helps you identify risks early and prepare comprehensive backup plans.
Backup Planning
Develop contingency plans tailored to AI-predicted scenarios. Focus on these three core areas:
1. Resource Allocation
Keep resources flexible by setting clear action thresholds. Reserve 10-15% of your operating budget as a strategic buffer for quick responses to unexpected situations.
2. Operational Adjustments
Prepare detailed protocols for each potential scenario. Clearly document actions, timelines, and responsibilities for various outcomes to ensure readiness.
3. Communication Protocols
Set up clear communication channels to share updates and coordinate responses across teams. Regularly schedule scenario review meetings with stakeholders to maintain alignment.
For each scenario, create specific action plans that include:
- Trigger conditions that activate the plan
- Necessary resources and budget allocations
- A timeline for execution
- Metrics to measure success and procedures for monitoring progress
Summary
Using AI-driven scenario planning effectively involves a detailed process that includes preparing data, configuring models, and applying insights in real-world contexts. Start with reliable historical data and establish clear response triggers for different time frames.
Here are some key practices to follow:
- Validate and clean your data
- Track short-term metrics to ensure accuracy
- Compare AI-generated forecasts with past trends
- Regularly update and refine your models
- Keep resources adaptable to changing needs
These steps align with the earlier guidance on data preparation and model training and work seamlessly with advanced FP&A systems.
Phoenix Strategy Group's financial modeling platform simplifies this process with automated data engineering and advanced analytics. Their FP&A systems enable real-time scenario tracking and adjustments, while their fractional CFO services provide expert support in interpreting AI insights to guide strategic decisions.
"As our fractional CFO, they accomplished more in six months than our last two full-time CFOs combined. If you're looking for unparalleled financial strategy and integration, hiring PSG is one of the best decisions you can make."
– David Darmstandler, Co-CEO, DataPath
To stay ahead, regularly evaluate your model’s performance, adjust trigger conditions, and fine-tune your response strategies.