How Predictive Insights Improve Financial Forecasting
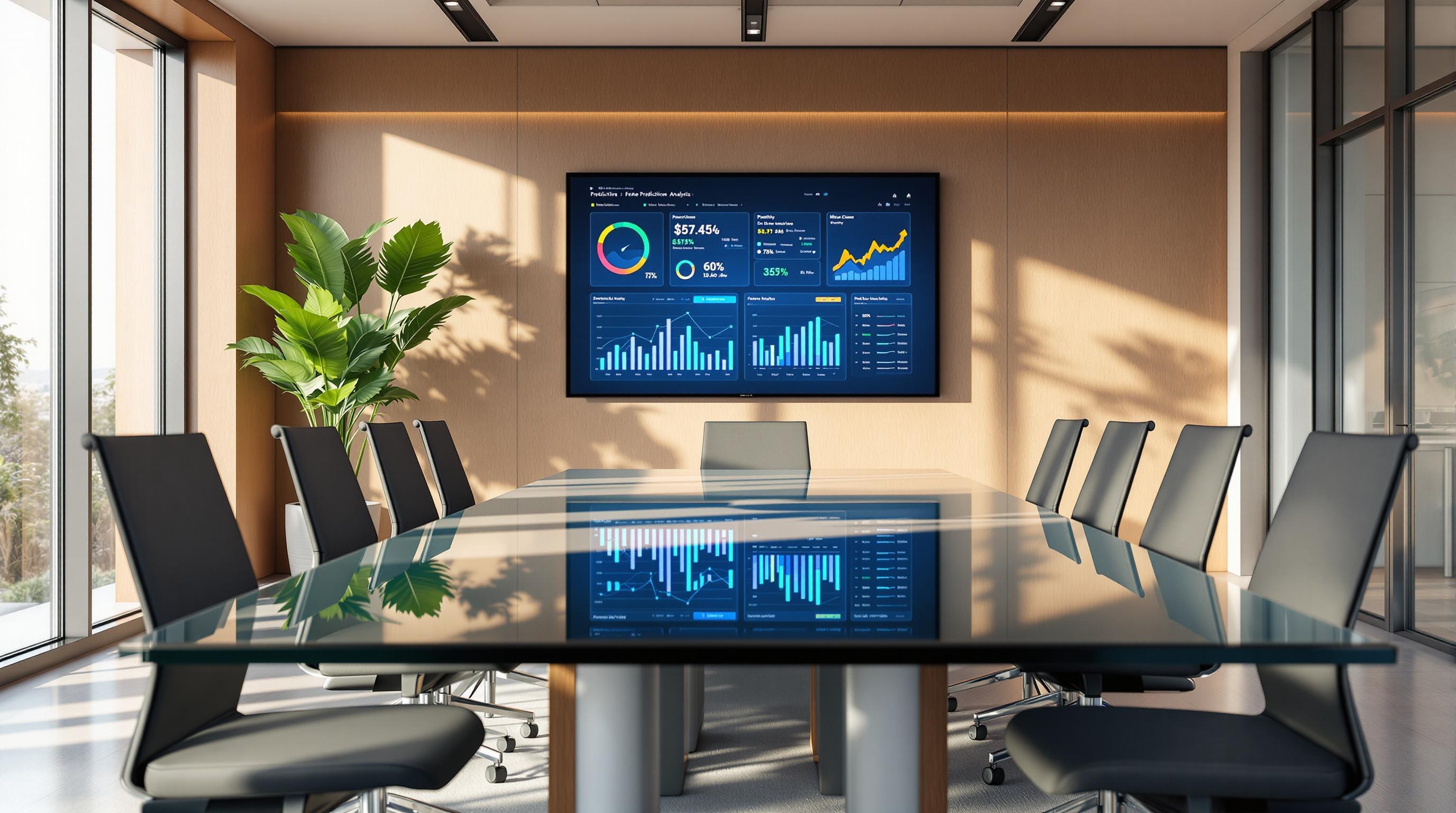
Predictive analytics is changing financial forecasting by making it more accurate and actionable. Businesses using these tools see 10-20% better forecast accuracy, reduce risks, and save time. For example, a bank cut loan defaults by 20% using predictive models, and a retailer lowered inventory costs by 15% while boosting sales by 2%.
Key Benefits:
- Better Accuracy: Detects patterns traditional methods miss.
- Risk Detection: Flags potential issues early.
- Real-Time Updates: Adjusts to market changes instantly.
- Efficiency: Reduces manual work and errors.
Challenges It Solves:
- Over-reliance on past data.
- Lack of real-time insights.
- Poor data integration.
- Time-consuming manual processes.
Predictive analytics uses diverse data sources (financial records, market trends, alternative data) and integrates them into dashboards for smarter decision-making. Companies like Phoenix Strategy Group help businesses adopt these tools quickly and effectively, offering a competitive edge in today’s market.
How Predictive Analytics Transforms Financial Forecasting
Predictive analytics is reshaping financial forecasting by revealing patterns that traditional methods often overlook. By leveraging advanced statistics and machine learning, it helps businesses make smarter, data-driven decisions rather than relying on intuition.
Understanding Predictive Insights
Traditional forecasting methods typically depend on past performance, but predictive analytics goes further. It processes large volumes of data to uncover hidden patterns and relationships. By combining historical financial records, market trends, and real-time data, it provides more precise future predictions.
For example, one major bank managed to cut loan defaults by 20% in just a year. How? By using predictive analytics to evaluate credit risks. The system analyzed factors like customer payment histories, market conditions, and economic indicators, enabling the bank to flag high-risk applications before they became issues.
This kind of insight paves the way for major improvements in financial forecasting.
Benefits of Using Predictive Analytics in Forecasting
Incorporating predictive analytics into financial forecasting offers a range of advantages:
Benefit | Impact |
---|---|
Improved Accuracy | Delivers 10-20% better precision by recognizing patterns in large datasets |
Early Risk Detection | Spots potential financial risks before they escalate into larger problems |
Real-time Adjustments | Automatically updates models in response to market changes |
Resource Efficiency | Simplifies baseline forecasts, saving time and reducing manual work |
To unlock these advantages, businesses need access to diverse, high-quality data sources.
Data Sources for Predictive Financial Models
Modern predictive models rely on a mix of data sources to create accurate forecasts. These include internal financial records (like sales and operational metrics), market intelligence (such as competitor performance and industry trends), and alternative data (like social media sentiment or satellite imagery).
"In the world of modern finance, data is no longer just an asset – it is the backbone of decision-making, strategy, and growth."
Companies can team up with experts like Phoenix Strategy Group to integrate alternative data into their predictive models. Success depends on combining varied data sources while ensuring their quality, creating a strong foundation for precise and actionable forecasts.
Using Predictive Insights in Financial Dashboards
Building Dashboards with Predictive Analytics
Modern financial dashboards must strike a balance between being easy to use and offering in-depth analysis. Interactive visuals help spotlight trends, while drill-down tools allow users to explore the details. For example, a mid-sized manufacturing company used predictive dashboards to fine-tune production scheduling. This approach led to a 25% drop in downtime and an 8% boost in throughput by accurately predicting maintenance needs and resource demands.
That said, even the most sophisticated dashboards are only as good as the data and predictive models they rely on.
Maintaining Data Quality and Model Accuracy
The quality of your data has a direct impact on how reliable your forecasts are. Here's how top organizations ensure their data and models meet high standards:
Component | Best Practice | Impact |
---|---|---|
Data Validation | Automated checks for missing values and outliers | Cuts errors by up to 40% |
Model Updates | Regular recalibration using fresh data | Keeps accuracy above 95% |
Quality Control | Routine audits of data sources and integrations | Ensures consistent inputs |
Ongoing validation, including monitoring performance and adjusting parameters, ensures predictions stay accurate and useful. While maintaining data quality is vital, choosing the right tools and expertise also plays a big role.
Tools and Experts for Dashboard Integration
After securing high-quality data, the next step is integrating predictive insights into your dashboards effectively. Companies like Phoenix Strategy Group specialize in building advanced, user-friendly dashboards by combining financial modeling with data engineering.
Businesses that work with professional integration services often see a 10-20% improvement in forecast accuracy compared to handling it in-house. This improvement comes from selecting the right tools, expert setup, and continuous model optimization.
When implementing dashboards, it's important to focus on:
- System scalability and flexibility
- Real-time data processing
- Customization options
- Built-in quality assurance features
Addressing Barriers to Predictive Analytics Adoption
Breaking down barriers is key to getting the most out of predictive insights in financial dashboards.
Combining Data from Different Sources
Bringing together data from various systems is one of the toughest challenges in using predictive analytics for financial forecasting. Many organizations face issues integrating data from ERP platforms, CRM software, and accounting tools. A centralized data warehouse can act as a single source of truth, but it needs a strong ETL (Extract, Transform, Load) process to clean, transform, and unify data formats across systems.
Key elements for effective data integration include:
Component | Purpose | Impact on Forecasting |
---|---|---|
Automated ETL Pipeline | Ensures regular data updates | Keeps forecasts current daily |
Data Validation Rules | Catches errors and standardizes formats | Cuts errors by 35% |
Once the integration issues are resolved, businesses face a critical choice: develop predictive analytics capabilities internally or collaborate with external experts.
Choosing Between In-House Expertise and External Partners
Deciding whether to build in-house or work with external partners depends on several factors:
Factor | In-House Development | External Partnership |
---|---|---|
Time to Implementation | 6-12 months | 1-3 months |
Cost Considerations | High upfront costs ($150,000-300,000) plus ongoing expenses | Lower upfront costs ($50,000-100,000) with maintenance included |
Expertise Level | Limited to internal team | Access to specialized expertise |
For example, Phoenix Strategy Group’s data engineering team can deliver predictive analytics solutions in weeks by leveraging their infrastructure and expertise.
Balancing Automation with Human Input
Automation can make processes faster, but human judgment remains essential for financial forecasting. The goal is to create a system where predictive models support human decisions rather than replace them.
This hybrid model involves experts validating assumptions and adjusting parameters during model development, analysts interpreting results and adding qualitative insights during operations, and continuous refinement through feedback. This approach typically improves forecast accuracy by 30-40% compared to relying solely on automation or manual methods.
sbb-itb-e766981
Measuring the Impact of Predictive Analytics on Forecasting
Let's break down the metrics and examples that highlight the impact of predictive analytics on forecasting.
Metrics for Evaluating Forecast Accuracy
Financial teams rely on specific metrics to gauge how well predictive models perform. These metrics combine statistical measures with business outcomes:
Metric Type | Key Measures | Target Accuracy |
---|---|---|
Statistical Accuracy | Mean Absolute Error (MAE), Mean Absolute Percentage Error (MAPE) | MAPE < 10% |
Financial Performance | Revenue Growth Rate, Profit Margins, ROI | Improvement > 15% |
Operational Efficiency | Cash Flow Accuracy, Inventory Turnover | Variance < 5% |
These metrics not only validate the models but also showcase their impact on real-world business scenarios, as shown in the example below.
Examples of Success with Predictive Forecasting
In the banking industry, one leading institution transformed its credit risk assessment using predictive analytics. Within just one year, they achieved a 20% drop in loan defaults. This result highlights how data-driven forecasting can improve risk management in measurable ways.
Improving Models with Feedback Loops
Feedback loops play a critical role in keeping predictive models accurate and effective. Here's how the process works:
Phase | Action | Impact |
---|---|---|
Data Collection | Gather actual vs. predicted outcomes | Spot deviations in patterns |
Analysis | Compare forecast accuracy over time | Identify model weaknesses |
Adjustment | Update model parameters and inputs | Enhance future predictions |
Organizations that use feedback loops often see a 30-40% boost in forecast accuracy compared to those relying on static models.
The team at Phoenix Strategy Group stresses the importance of blending automated data tools with expert financial analysis. This combination ensures predictive models stay precise and aligned with business goals.
Conclusion: Predictive Insights as a Tool for Growth
Summary of Key Points
Predictive analytics has transformed financial forecasting, shifting it from a reactive process to a forward-thinking strategy. The International Institute of Forecasters reports that companies using predictive analytics see 10-20% greater forecast accuracy compared to older methods. For example, a major bank used predictive analytics for credit risk assessment, analyzing over 100 variables. The result? A 25% drop in default rates within just 18 months - proof of how data-driven decisions can deliver real results.
These benefits highlight the potential for businesses to integrate predictive analytics into their operations and make smarter, more informed decisions.
Steps for Businesses to Get Started
Creating a predictive analytics program requires careful planning and execution. Phoenix Strategy Group advises businesses to focus on two key areas: strong data governance and a reliable technology infrastructure. Collaborating with experts who bring both technical skills and industry knowledge can help ensure data is collected, analyzed, and used effectively - all while maintaining high standards for data quality and security.
The market for predictive analytics is projected to grow from $20.5 billion to $30 billion by 2028, with an annual growth rate of 20.4%. This growth highlights its increasing importance in improving financial forecasting and driving business success. Companies that adopt predictive analytics now can gain a competitive edge through precise, data-backed financial planning and smarter decision-making.
FAQs
What does a financial dashboard look like?
A financial dashboard combines live data with forecasts, using clear visuals to help teams make decisions faster. These dashboards typically include three main parts:
Component | Description | Key Elements |
---|---|---|
Performance Metrics | Current financial status | Revenue trends, cash flow, profit margins |
Predictive Indicators | Future-focused insights | Forecast models, risk alerts, trend predictions |
Interactive Elements | Tools for deeper analysis | Drill-down options, scenario modeling |
For instance, a logistics company used predictive dashboards to fine-tune delivery routes. This reduced transportation costs by 12% and boosted on-time deliveries by 18%. By combining real-time data with predictive insights, they overcame the limits of traditional forecasting and made smarter, faster decisions.
"High-quality data is the foundation of effective financial dashboards, ensuring predictive models deliver accurate and actionable insights. This involves gathering, cleaning, and organizing financial data while leveraging advanced algorithms to detect patterns."
Good dashboards strike a balance between being easy to understand and offering in-depth analysis. They often include KPI scorecards to track both past results and future forecasts, giving finance teams the tools they need to tackle challenges and seize new opportunities.